Undergraduate Research at Jefferson Lab
Machine-Learning PID for SOLID Detector Electromagnetic Calorimeter Beam Test
Student: Darren Upton
School: University of Virginia
Mentored By: Xiaochao Zheng
The Solenoidal Large Intensity Device SoLID seeks to fill the need for a large-acceptance, high-luminosity spectrometer in Hall A of Jefferson Lab. In order to characterize the electromagnetic calorimeter modules for SoLID, the collaboration has conducted a beam test study to validate our hardware. A critical component of this study is establishing SoLID's ability to distinguish between different particles, or particle identification PID. During this beam test, we have worked to bridge the gap between simulation and data in order to improve the accuracy of GEANT-based simulation and provide insight into analyzing our data. By accomplishing this, we explore traditional PID methods, as well as machine-learning PID methods for analyzing beam test data. During benchmarking of PID performance using simulation, we show that ML algorithms outperform traditional cuts with higher electron efficiency and pion rejection for a range of momentum bins and trigger settings. We additionally show ML PID methods allow for complex combinations of models trained for individual detectors without the characteristic loss in efficiency associated with traditional methods. After further validation, we seek to apply ML and traditional PID methods to beam test data to complete the proof of concept and laid foundational work for ML PID methods for a fully-realized SoLID.
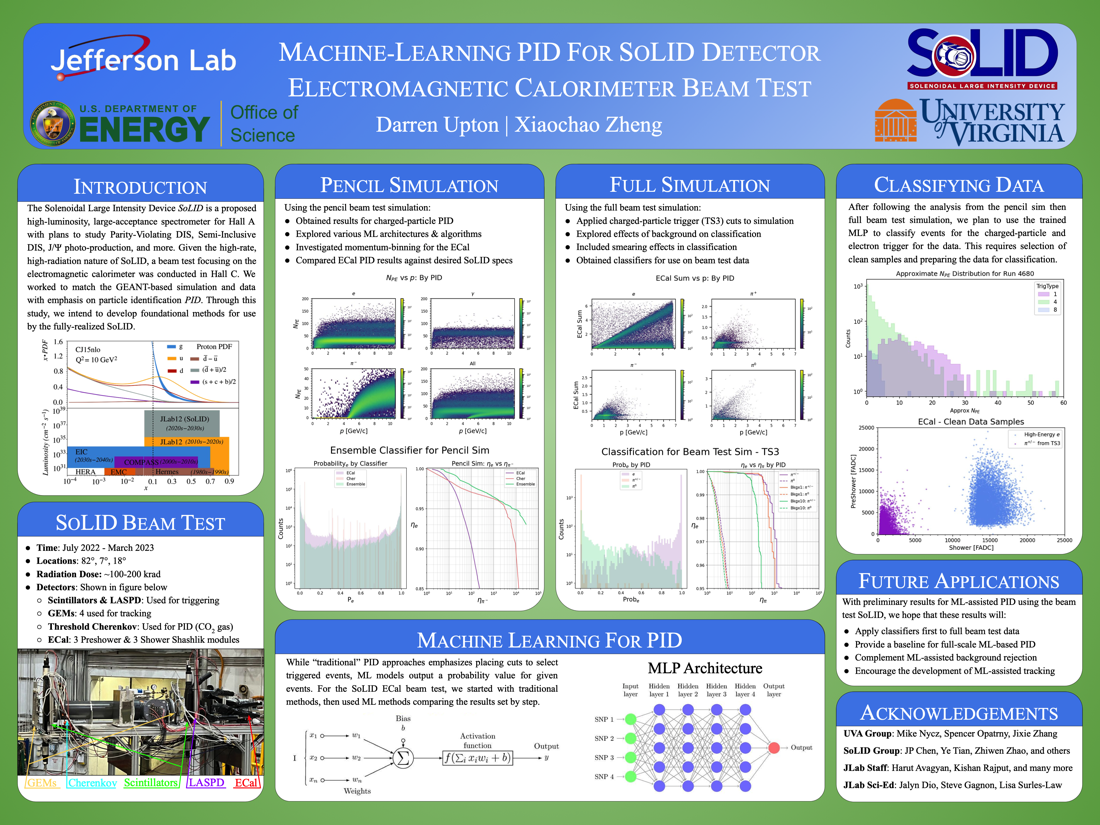
Citation and linking information
For questions about this page, please contact Carol McKisson.