Undergraduate Research at Jefferson Lab
Machine Learning in Accelerator Corrections: FFA Multipass Variability
Student: Annika Tomtschik
School: Oklahoma Baptist University
Mentored By: Ryan Bodenstein, Alexander Coxe, Kirsten Deitrick and Donish Khan
Design studies are underway for an upgrade to 20-24 GeV at the Continuous Electron Beam Accelerator Facility (CEBAF) at Jefferson Lab. The cornerstone of this upgrade is fixed field alternating-gradient (FFA) magnet arcs which recirculate an electron beam with multiple energies through the accelerator. Position and optics corrections in FFA arcs are complex and typically use linear controls; an optimized machine learning routine may do better. This project tested various neural networks to evaluate efficacy for the FFA upgrade to CEBAF. 'TensorFlow' and 'Keras' packages were used to construct neural networks which were evaluated on data from a toy model of a proportional derivative (PD) controller. The intuition gained from the toy model was used to estimate performance on simulated accelerator dynamics. It was shown that Long/Short Term Memory (LSTM) layers were useful for beam correction. The neural network model proved to be as or more effective than singular value decomposition (SVD) for a single pass in the accelerator. Thus, machine learning could lead to a more effective correction scheme and minimize the effects of beam errors on experiments.
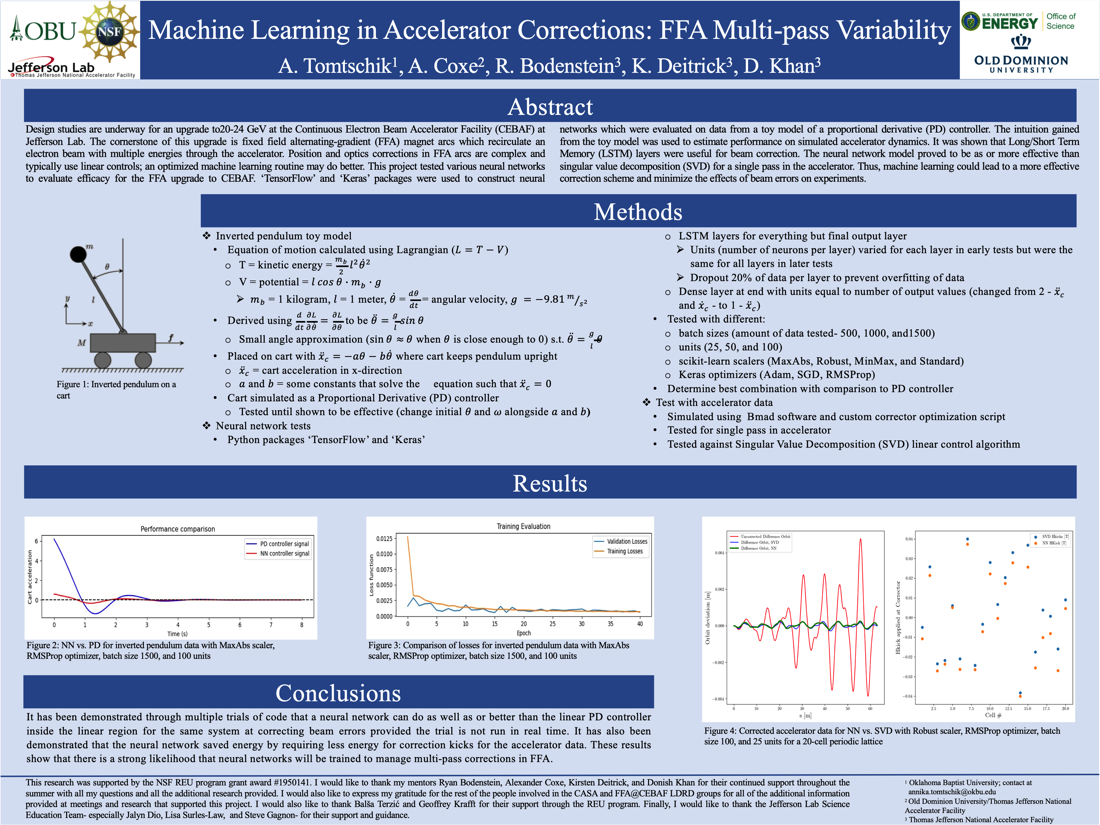
Citation and linking information
For questions about this page, please contact Education Web Administrator.